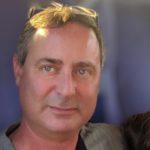
In response to demands from other African languages, he developed a model for multilingual lexicography through which languages interlink at a fine-grained semantic level. These knowledge-based relations undergird underfunded translation technologies he is currently building for all 7111 ISO-coded languages.
Among his writings, he is the author of “Teach You Backwards: An In-Depth Study of Google Translate for 108 Languages“, an empirical investigation of Google’s results in the context of larger questions pertaining to the enterprise of Machine Translation. His lab is now seated at the Swiss EdTech Collider at EPFL in Lausanne.
Abstract
How AI Cured Coronavirus and Delivered Universal Translation, and Other MT Myths and Magic
Artificial Intelligence, Neural Networks, and Machine Learning are often viewed as the launchpad to the future throughout the information technology industry. Research in language technology, especially Machine Translation, has steered increasingly toward these topics. The popular press magnifies the enthusiasm, leading many in the public to believe that universal translation has arrived, or is just around the corner. To what extent is this enthusiasm warranted?
AI is eminently suited for some tasks, and ill-fitted for others. Through analysis of mounds of weather data, for example, AI can detect patterns and make predictions far beyond traditional observational forecasts. On the other hand, an AI-based dating app would surely fail to connect would-be lovebirds beyond a few of the sci-fi faithful, because finding a match involves far too many variables that cannot be constituted as operable data.
Within language technology, tasks that are suitable for AI include finding multiword expression (MWE) candidates, or learning patterns that can predict grammatical transformations. Unsuitable tasks include deciding what word clusters are legitimate MWEs, or how underlying ideas could be effectively rendered in other languages without vast troves of parallel data. Yet the success, or hint thereof, for AI to achieve some Natural Language Processing (NLP) tasks has been frequently transmogrified as near-mastery of translation by computer science.
The assumptions about what AI can do for MT are built on several myths:
- We have adequate linguistic data. In fact, well-stocked parallel corpora are just part of the journey, and only exist for a smattering of language pairs at the top of the research agenda, not for the vast majority. By contrast, little or no useful digitized data exists for most of the world’s languages that are the mother tongues of most of the world’s people.
- Neural Networks have conquered the barriers faced by previous MT strategies, at least for well-provisioned languages. In fact, we can see recent qualitative improvements for certain languages in certain domains, but even the best pairs fail when pushed beyond the zones for which they have comparable data. Weather forecasts can be perfectly translated among numerous languages, for example, while seductive conversations for online dating will break MT in any language.
- Machine Learning yields ever more accurate translations. In fact, MT almost never channels its computed results through human verification, so it cannot learn whether its output is intelligible. We thought computers had learned to recognize gorillas from people tagging “gorilla” to a myriad of images, until we found machines applying that learned label to dark-skinned humans as well. Learning a language is lot more complicated than learning to recognize a gorilla. People learn languages through years of being informed and corrected by native speakers (parents, teachers, friends), and adjusting their output when they fail to be understood. For language learning by computers, the same iterative attention is no less necessary./li>
- Zero-shot translation bridges languages that do not have parallel data connections. In fact, zero-shot is purely experimental and the numbers so far are rock bottom.
Although AI is still in its infancy for translation and other NLP, we know its nutritional needs to grow toward maturity: data. This talk will conclude by describing two methods for collecting the data to power future MT. The first is already viable, using crowds to play games to provide terms for their languages, aligned semantically to a universal concept set. This method dispenses with the computational inferences about how words map across languages, in favor of natural intelligence. Such data can provide the bedrock vocabulary, including inflections and MWE’s, that hard-wired linguistic models and neural networks can then use to achieve grammatically and syntactically acceptable equivalence in other languages within the system.
The second method is still in vitro. With vocabulary data in place, users can currently tag their intended sense of a word or MWE on the source side, constricting translations to terms that share the same sense. Those tags can become a rich vein of sense-disambiguated data from which machines can truly learn. AI cannot now translate among most languages, in most contexts, but, with sufficient well-specified, well-aligned data, it could.